Theme 1: Freight Data Warehouse (FDW), Data Collection and Data Science Applications
Theme 1: Freight Data Warehouse (FDW), Data Collection and Data Science Applications
The FDW provides storage and access to large freight datasets necessary for the execution of CLUE research projects. The data enables novel data science applications, including applications in Theme 1 on freight flows, bottlenecks, safety and fuel efficiency, and pandemic supply chain performance and several projects in Themes 2 to 4. Two surveys and several more smaller scale surveys are conducted to enable analysis of freight demand.
Project Leaders
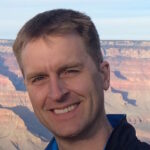
Dr. Matthew RoordaProfessorDepartment of Civil & Mineral Engineering - University of Toronto
Projects:
Project 1.1: Establish the Freight Data Warehouse (FDW)
Project 1.4: Applying Data Fusion for Freight Bottleneck Analysis
Project 1.6: Supply Chain Analytics During/After Pandemic
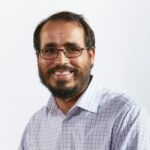
Dr. Elkafi HassiniProfessorDegroote School of Business, McMaster University
Projects:
Project 1.3: Visualizing and Animating Telematics Data
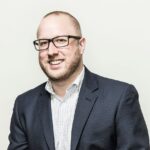
Dr. Kevin Gingerich
Assistant ProfessorCivil Engineering, York University
Projects:
Project 1.2: Shipper and Truck Driver Surveys
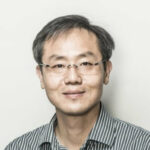
Dr. Peter Park
Associate ProfessorCivil Engineering, York University
Projects:
Project 1.5: Assessing Network Safety and Fuel Efficiency via Big Data Analytics
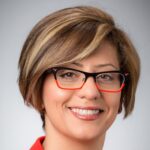
Dr. Saiedeh Razavi
Associate ProfessorCivil Engineering, York University
Projects:
Project 1.3: Visualizing and Animating Telematics Data
Partners
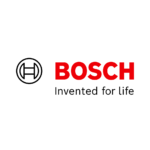
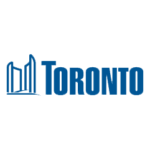
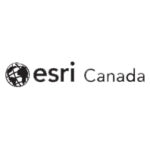
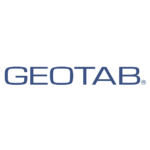
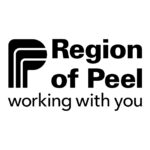
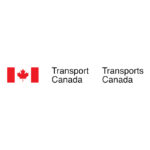
Projects
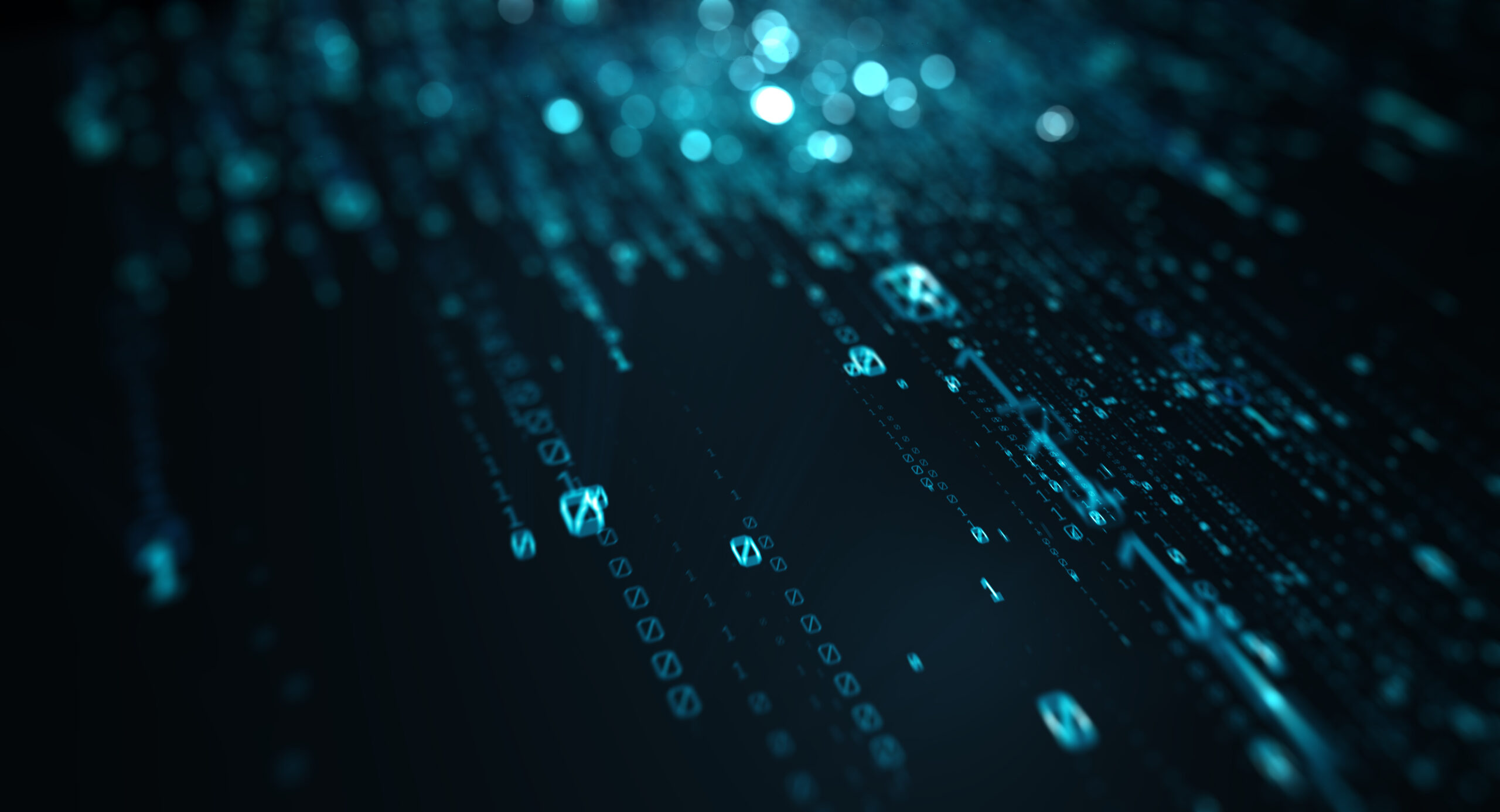
Project 1.1: Establishing the Freight Data Warehouse (FDW)
Led by Dr. Matthew Roorda
University of Toronto
Research Plan: The Smart Freight Centre (SFC) provides secure freight data storage, data management and visualization services to CLUE participants, thereby enabling research of all project participants while protecting confidentiality and respecting data sharing agreements and ethics protocols. The FDW leverages telematics, speed and flow data from Here, ATRI and Shaw/Omnitracs via Transport Canada, data from other partners and survey data from various projects. The FDW is hosted on the University of Toronto Private Cloud to enhance a visualization-enhanced secure data platform. Integrity and security of the data is ensured via access protocols that reflect data-sharing agreements, ensure cybersecurity and preserve confidentiality. A remote data querying system is developed and maintained, and an appropriate approval mechanism is developed to ensure appropriate oversight of the distribution of data. Visualization capabilities are developed such that the outcomes of Theme 1 projects can be disseminated via the querying system.
Project Goal: State-of-the-art freight data service for the GTHA.
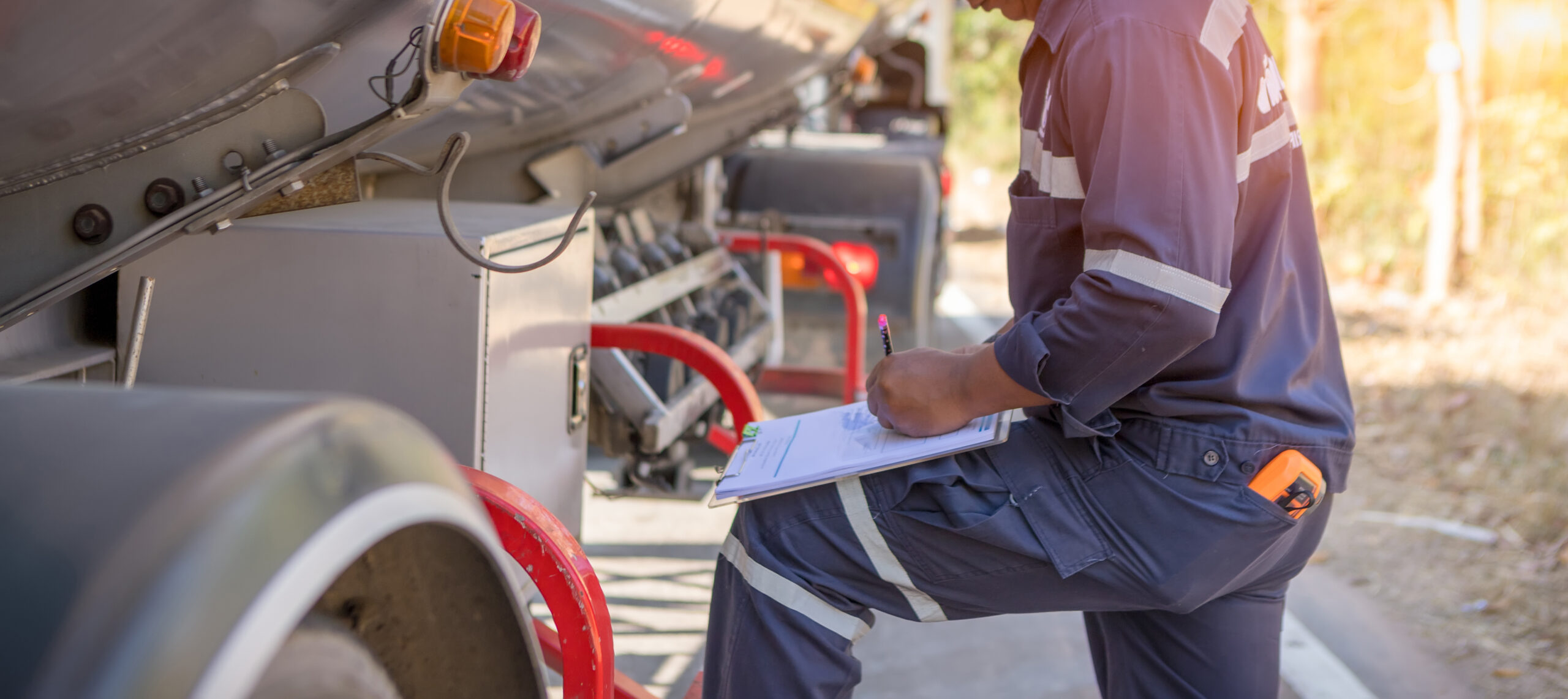
Project 1.2: Shipper and Truck Driver Surveys
Led by Dr. Kevin Gingerich
York University
Research Plan: Freight data are essential for analyzing freight demand, developing freight forecasting models and assessing patterns of truck movements. This project designs and executes two major surveys, including one with 1000 shipper establishments, and another with 1000 truck drivers. The shipper survey captures truck trip generation, vehicles owned, commodities shipped, shipment destinations, and company information. The truck driver survey collects data about preferred truck corridors and rest stops, technology adoption, travel delay, and costs.
Project Goal: This project establishes characteristics of freight activity to be used by engineers to estimate freight trip generation and planners to forecast future freight activity. Recent trends in the industry are also of interest to the general public.
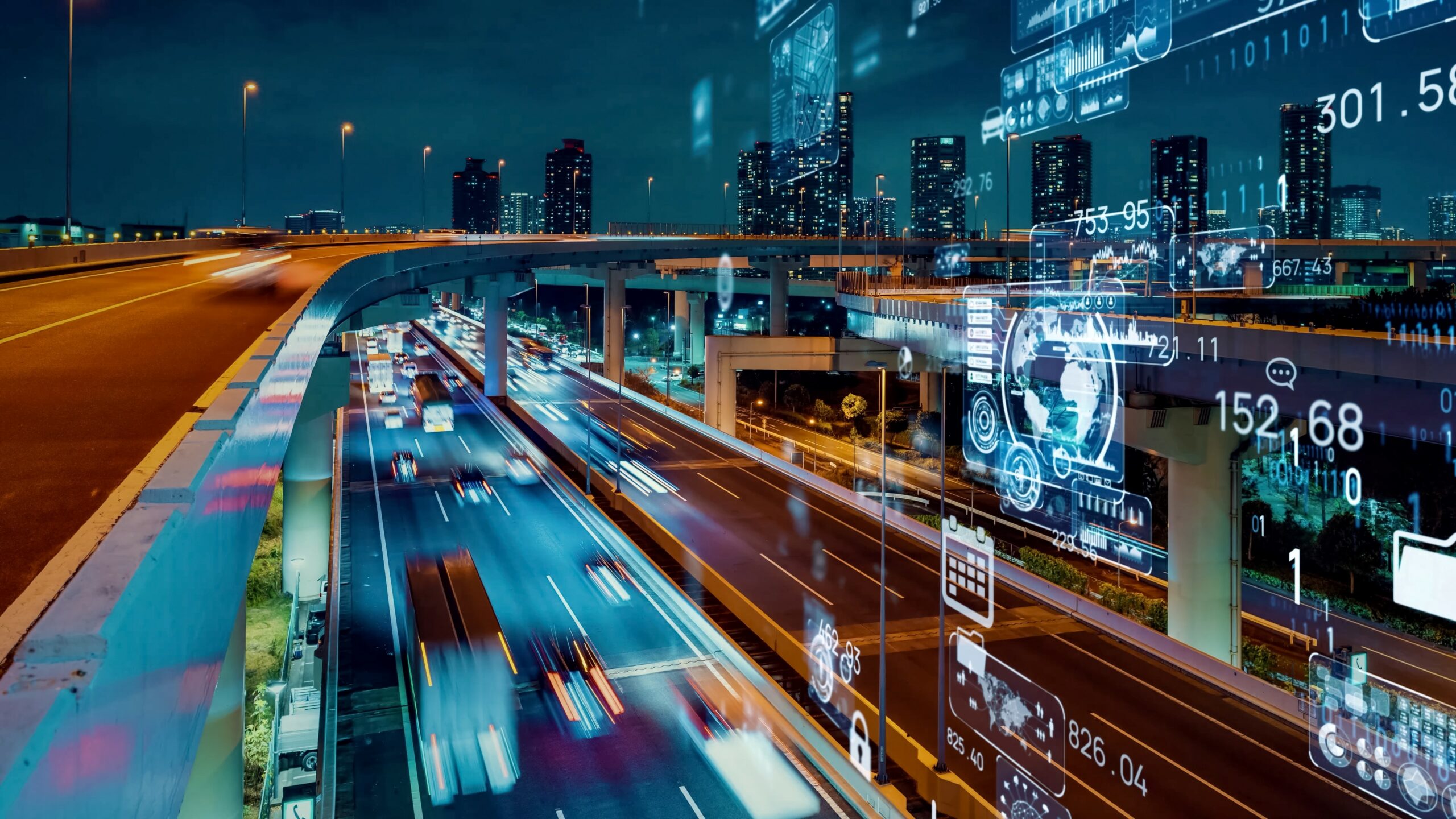
Project 1.3: Visualizing and Animating Telematics Data
Led by Dr. Elkafi Hassini & Dr. Saiedeh Razavi
McMaster University
Research Plan: To develop visualization applications to provide new insights into freight flows and types, travel speeds, traffic congestion, and economic throughput of freight transportation corridors. This data science application project emphasizes big data processing, data imputation and gap-filling, and augment traditional visualization techniques with animation and non-speech audio. Data is obtained from the FDW, as well as our partners such as Peel Region and Geotab. We are collaborating on visualization with Esri Canada and leveraging existing high-performance computing infrastructure and software in the MiSCAN lab at McMaster University, which makes visualization outcomes available on the FDW.
Project Goal: Visualization applications for freight flow and traffic congestion in urban centres that can be used by local government to develop land zoning and economic development plans.
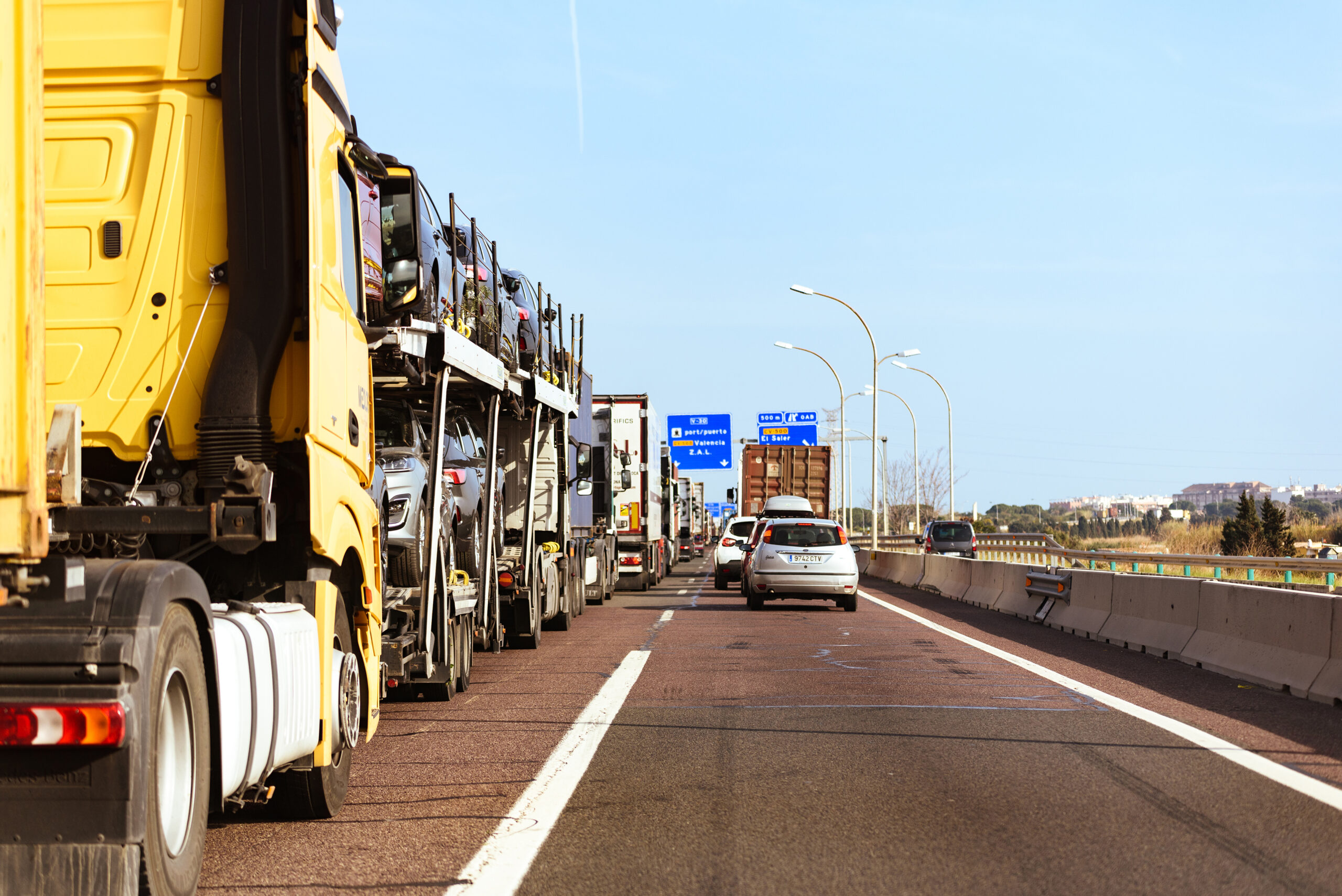
Project 1.4: Applying Data Fusion for Freight Bottleneck Analysis
Led by Dr. Matthew Roorda
University of Toronto
Research Plan: This project develops and applies novel data science techniques to identify industry-specific economic impacts of freight caught in traffic bottlenecks. Data fusion techniques are developed to integrate telematics and engine data (which do not give information about commodity or truck type) with survey and roadside interview data (which provide commodity, origin and destination, and truck type information, but do not have detailed spatio-temporal information).
Project Goal: Provide evidence-based policy support to government partners to address freight bottlenecks in the GTHA and enhance performance of key industry sectors.
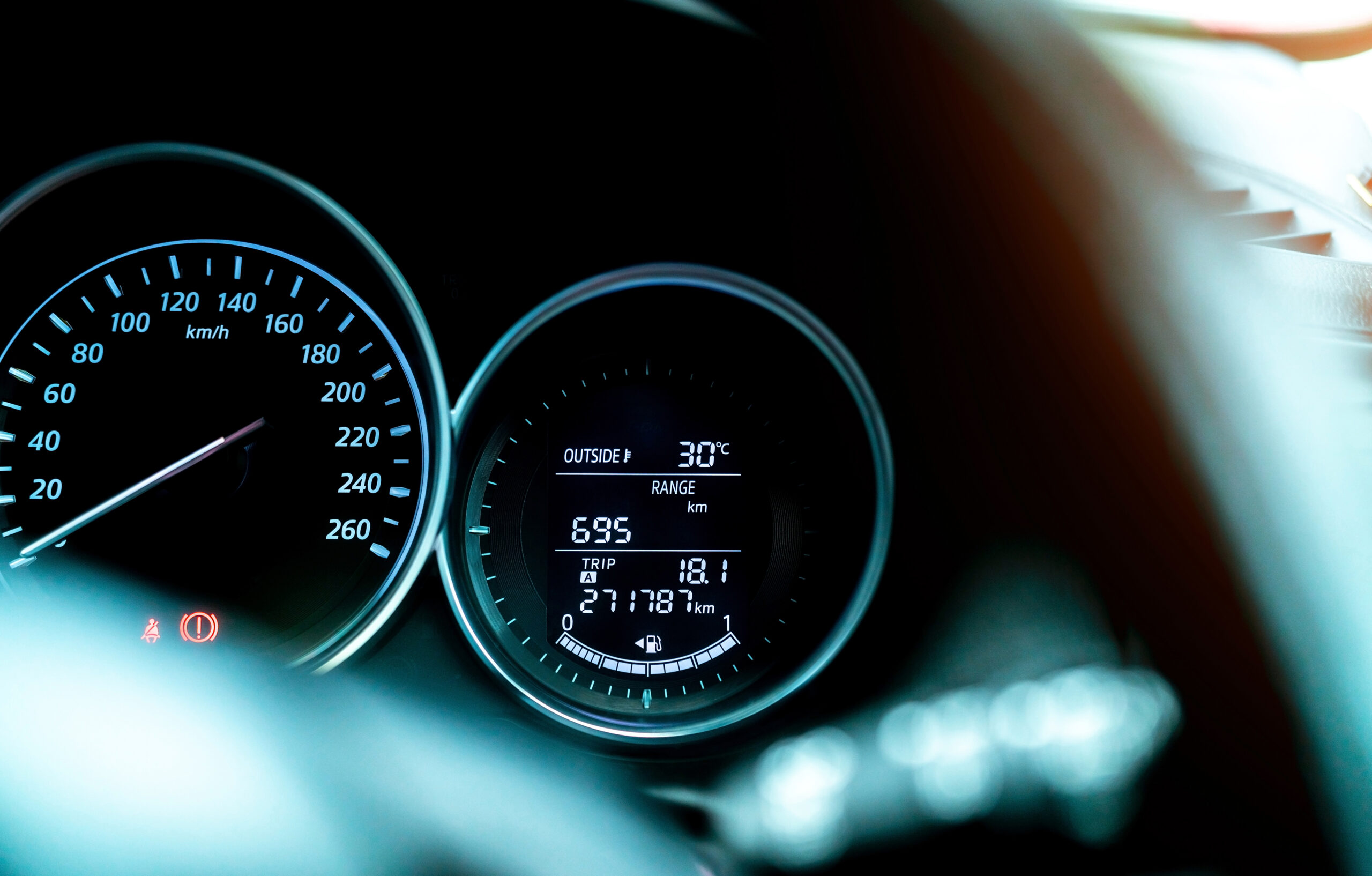
Project 1.5: Assessing Network Safety and Fuel Efficiency via Big Data Analytics
Led by Dr. Peter Park
York University
Research Plan: This project combines FDW and Geotab truck engine and telematics data with data on collisions and weather conditions to develop novel network screening methods for assessing GTHA road network performance in terms of safety and fuel efficiency considering weather impact. Telematics data are used, such as travel speed and flow data from Here, ATRI and Shaw/Omnitracs via Transport Canada. The telematics data from Geotab also includes micro-level information such as hard-braking, fast acceleration and high-speed cornering which indicates the driving behaviour of individual truck drivers. The data is combined with collision data from police reports, and detailed weather data from Environment Canada. Data from surveys conducted in other projects are also used in this project. Parametric methods (e.g., Bayesian fusion) and non-parametric methods (e.g., machine learning) are applied to find relationships between truck driving behaviour, traffic flow, weather conditions, roadway geometrics, and collision rates.
Project Goal: Analysis for the GTHA and state-of-the-art network screening processes.
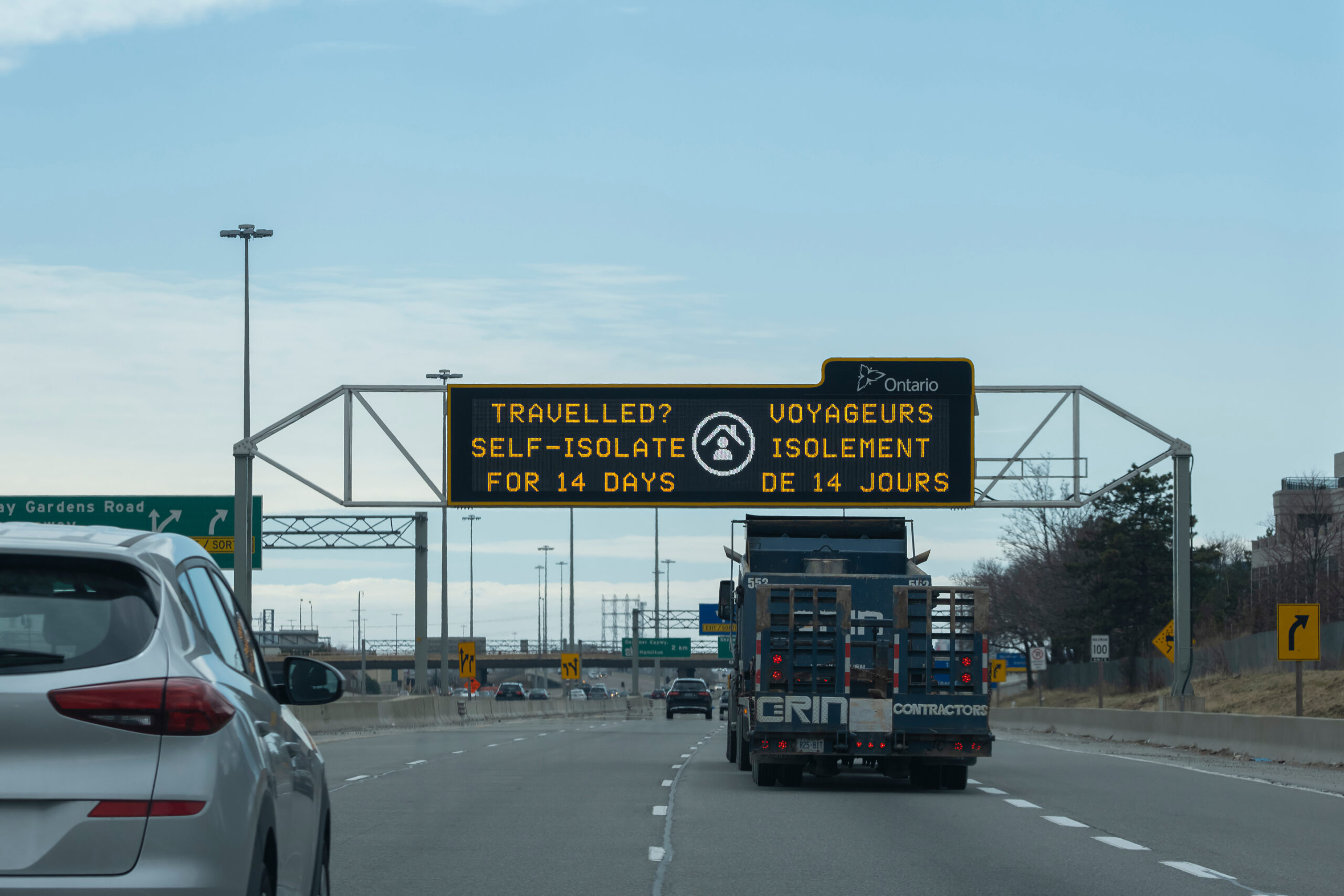
Project 1.6: Supply Chain Analytics During/After Pandemic
Led by Dr. Matthew Roorda
University of Toronto
Research Plan: This project uses a variety of datasets to understand the impacts of COVID-19 on the supply chain, monitor the return to normal operating conditions, and develop lessons learned about supply chain resilience in Canada. Comparisons are being made on a monthly basis including travel speeds from Here, truck GPS data from ATRI, Shaw/Omnitracs, and Geotab, and qualitative experiences from Project 3.1 Off-peak Delivery Extended Pilot. This project attempts to show differences in average travel speeds, differences in the pattern of truck travel, by time of day and by location (considering greater needs for home delivery, less congestion, changes in consumer and business demand for products). As the pandemic unfolds, adjustments are made to the research questions to address the most pertinent supply chain concerns.
Project Goal: Evidence-based lessons learned for Canadian urban areas are developed post-pandemic, to help public and private sector partners better plan for such events in the future.